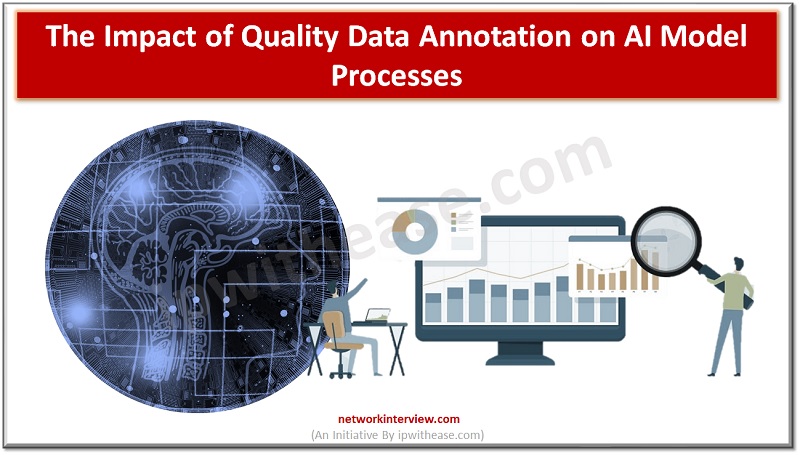
The Impact of Quality Data Annotation on AI Model Processes
Artificial intelligence (AI) models are now widely used in many applications, including natural language processing, autonomous cars, and medical diagnosis. These AI models mainly rely on data annotation, a vital step in supervised learning that includes labeling data. The caliber of data annotation directly impacts the efficacy and performance of AI model procedures. The relevance of high-quality data annotation is highlighted in this article.
Understanding Data Annotation
Data annotation labels unprocessed data to offer factual data to train AI models. AI models can learn from labeled data and generalize patterns to make precise predictions thanks to several data annotation techniques, including image, text, audio, and video annotations. AI models can recognize and comprehend ways by receiving annotated data, which improves performance and decision-making abilities. The reliability of the training data is ensured through high-quality data annotation, which is essential for creating effective and reliable AI models.
Impact of Quality Data Annotation
The effectiveness or failure of AI applications can be affected significantly by high-quality data annotation’s effects on model operations. The main ideas highlighting this impact are as follows:
Improved Model Performance
When trained on well-annotated data, AI models display improved skills to recognize patterns, make precise predictions, and effectively perform complicated tasks. AI models can serve better and produce better outcomes. This is where a data annotation company provides accurate and trustworthy solutions. Models can better generalize to new data when the noise and biases are reduced through good annotation. As a result, model performance is improved, errors are reduced, and AI applications are used more effectively overall.
Accuracy and Reliability
In developing AI models, accuracy and reliability are crucial characteristics. The accuracy of a forecast is the assurance that the model’s outputs correspond to the labels found in the real world. For practical applications, trustworthy and dependable AI models constantly deliver accurate results. High accuracy and reliability are attained by quality data annotation, which gives AI models the essential framework to successfully learn patterns, reduce errors, and make precise conclusions.
Generalization and Adaptability
When AI models are trained on thoroughly annotated data, they may efficiently generalize their knowledge to handle brand-new, untainted material. This capability makes AI models more robust and versatile by enabling them to perform effectively outside the training data in real-world circumstances. Quality annotations also help AI models adapt to shifting surroundings, allowing them to constantly advance and provide precise predictions in ever-changing and dynamic contexts.
Reduced Bias and Fairness
High-quality data annotation is essential to minimize bias and advance fairness in AI model operations. Developers can reduce unfair depictions of particular groups or demographics by carefully selecting and annotating data. Ethical data annotation procedures guarantee that AI models treat every person fairly, regardless of background. As a result, users are more likely to trust and accept AI models that are just and equal and make objective judgments. AI technologies that have a beneficial social impact and avoid perpetuating harmful preconceptions or discrimination have less bias and more fairness.
Efficient Learning
High-quality data annotation enables efficient learning by allowing AI models to recognize patterns and correlations in the annotated data quickly. Accurate annotations give the model clear and instructive signals that help it learn from instances. Fewer training iterations are needed for AI models with well-annotated data, which lowers training costs and speeds up learning. The model performs better overall in various applications and can produce accurate predictions due to efficient learning.
Minimizing Overfitting and Underfitting
In the training of AI models, high-quality data annotation reduces overfitting and underfitting. A model can learn relevant patterns using accurate image annotation services without memorizing the training data (overfitting) or missing important marks (underfitting). It guarantees that the AI model generalizes well to fresh, unexplored data, enhancing its dependability and utility in practical applications.
Effective Problem Solving
AI models can recognize and comprehend complicated patterns in the data by being given accurate and trustworthy annotations, which enables them to make wise decisions when presented with real-world problems. The ability of the model to solve problems is improved by having well-annotated data, which allows the model to provide pertinent and insightful solutions across various areas, making AI an essential tool for tackling complicated issues and fostering creativity.
Conclusion
In conclusion, there is no disputing the influence of high-quality data annotation on AI model operations. Annotations that are accurate and trustworthy improve model performance, increase generalization, and reduce biases, making AI models more responsible, efficient, and equitable. Utilizing the potential of high-quality data annotation opens the path for AI’s revolutionary effects in various industries.
Continue Reading:
Artificial Intelligence vs Machine Learning
Automation vs Artificial Intelligence: Understand the difference
Tag:New technology